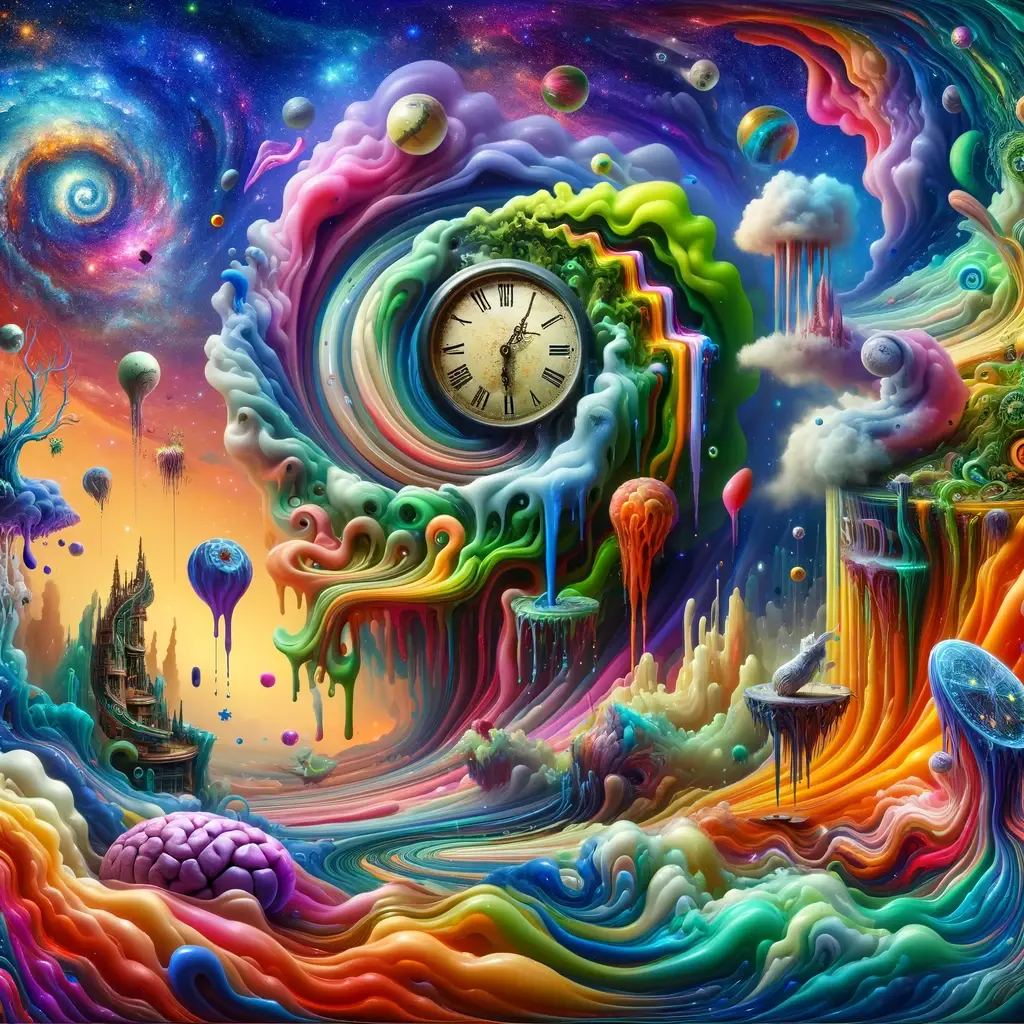
AI has undoubtedly done it’s fair share of changing industries due to it’s high-speed data processing, decision-making, and automation for multiple tools and uses. With that, there has also come about a situation where AI outputs are not grounded in reality or factual data. You’d see this in referenced statistics, citations, and more. This is known as AI hallucination and has emerged as a massive challenge for developers and users alike.
What is AI Hallucination?
An AI hallucination occurs when you add a script to an AI system and the output is something that’s completely fake. It’s a response not grounded in reality and based on false data. These responses can range from minor misses to an entire fiction book written by AI to prove its point. We don’t mean it’s a hallucination in the sense we’re usually familiar with. It’s a phrase used because the AI believes the output to be correct, as a human would believe their hallucinations to be correct, even if they’re misleading.
Causes of AI Hallucination
There could be a ton of reasons for an AI producing “hallucination-like” responses. One being that it’s an AI, haha, others are:
- Data Quality and Bias: Machine learning is based on different inputs and learning from those inputs. Considering this, there could be poor-quality data or biased datasets can lead to incorrect outputs.
- Model Limitations: There are and most likely will be for a very long time, limitations in the AI’s understanding and reasoning of data, logic, and inputs which will continue to lead to misinterpretations.
- Overfitting: When a dataset is too close to the AI’s training data, it could have a hard time figuring out what is new, what’s unseen, and then give a hallucination output.
Examples of AI Hallucinations
We’ve seen them before, but if you haven’t, look out for:
- Generating fictitious information in text generation models like GPT-3
- Producing incorrect translations in language models.
- Misidentifying objects in image recognition systems.
Mitigating AI Hallucinations
You can use a few strategies to work around the AI hallucinations:
- Improving Data Quality: Make sure your input datasets are accurate and representative. If they are, it can reduce the amount of biases and errors on output.
- Regular Auditing and Testing: Don’t just copy and paste everything you get back. Check the output so you can become familiar with the false information and then you could correct it or ignore it.
- Transparency and Explainability: Whenever you get your output, ask the model to explain its reasoning for the output. Sometimes it can help process and understand the actual path to the output and eliminate hallucinations.
- Human Oversight: Add human judgment to the decision-making process of your models. By doing this, you can catch and correct any of the hallucinations before you copy and paste them.
Takeaways
While AI has made significant strides, AI hallucinations show a massive challenge in terms of development and deployment. Understanding their causes and implementing a roadmap for avoiding them will help users and developers to avoid the hallucinations which will make AI a bit more reliable and eventually a more beneficial tool in various applications.
Ready To Scale Your Startup In 2024? Check Out Be Uniic's Free Audit Bundle Today!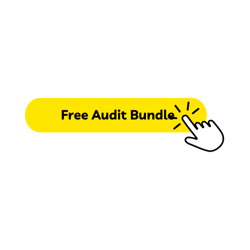